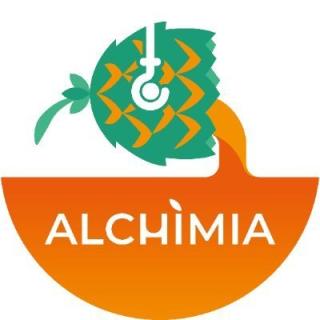
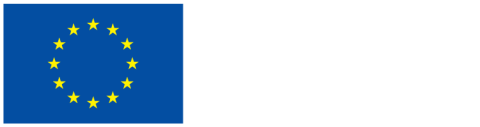
Energy-intensive industries (EIIs), embedded in many strategic value chains, make up more than half of the energy consumption of the European industry and reducing their CO2 intensity is crucial for meeting the objectives of the Paris agreement. Within EIIs, metallurgy poses a major challenge due to the trade-off that must be found between maintaining economic profitability, while progressively implementing the required transformations for a greener production. While digitalisation is generating a data deluge, Artificial Intelligence cannot be fully adopted due to limitations to share data between several factories and the heterogeneity of systems that hinders the replicability of AI.
ALCHIMIA aims to build a platform based on Federated Learning (FL) and Continuous Learning (CL) to help large European metallurgical industries unlock the full potential of AI to support the transformations needed to create high quality, competitive, efficient and environmentally friendly manufacturing processes.
The project will specifically address the challenges of the steel sector by creating an innovative system that automates and optimizes the production process dynamically with a holistic approach including scrap recycling and steelmaking.
As the name suggests, ALCHIMIA will find an optimal combination to reduce energy consumption, emissions and waste generation in the steelmaking process, while ensuring high quality products. The replicability and scalability of ALCHIMIA will be enabled through a complementary use case for the manufacturing of automotive parts. The developed system will be used for prognostic optimisation of the mix of input materials charged in the furnaces to obtain a certain product quality that matches the customers' specifications while reducing the environmental impact and energy consumption. ALCHIMIA will not only seek the optimal mix for the charge of metallurgy furnaces, but it will also determine the best combination of learning capacities to enable a smooth green transition for all industries thanks to unprecedented collaboration.
Atos is the project coordinator but also will have a strong technical contribution by defining the project technical specification and reference architecture.
Atos is also the leader of WP3 (Federated Learning and Continual Learning) to enable Machine Learning models to be trained in a distributed manner, avoiding the need to collect in a single and central repository data coming from multiple devices, machines, processes, systems and facilities. The resulting framework will be applied and demonstrated in two use-cases provided by Celsa Group and Fonderia di Torbole.